Expo
view channel
view channel
view channel
view channel
view channel
view channel
view channel
RadiographyMRIUltrasoundNuclear Medicine
Imaging ITIndustry News
Events
- Higher Chest X-Ray Usage Catches Lung Cancer Earlier and Improves Survival
- AI-Powered Mammograms Predict Cardiovascular Risk
- Generative AI Model Significantly Reduces Chest X-Ray Reading Time
- AI-Powered Mammography Screening Boosts Cancer Detection in Single-Reader Settings
- Photon Counting Detectors Promise Fast Color X-Ray Images
- AI-Powered MRI Technology Improves Parkinson’s Diagnoses
- Biparametric MRI Combined with AI Enhances Detection of Clinically Significant Prostate Cancer
- First-Of-Its-Kind AI-Driven Brain Imaging Platform to Better Guide Stroke Treatment Options
- New Model Improves Comparison of MRIs Taken at Different Institutions
- Groundbreaking New Scanner Sees 'Previously Undetectable' Cancer Spread
- Novel Radiotracer Identifies Biomarker for Triple-Negative Breast Cancer
- Innovative PET Imaging Technique to Help Diagnose Neurodegeneration
- New Molecular Imaging Test to Improve Lung Cancer Diagnosis
- Novel PET Technique Visualizes Spinal Cord Injuries to Predict Recovery
- Next-Gen Tau Radiotracers Outperform FDA-Approved Imaging Agents in Detecting Alzheimer’s
- Smart Ultrasound-Activated Immune Cells Destroy Cancer Cells for Extended Periods
- Tiny Magnetic Robot Takes 3D Scans from Deep Within Body
- High Resolution Ultrasound Speeds Up Prostate Cancer Diagnosis
- World's First Wireless, Handheld, Whole-Body Ultrasound with Single PZT Transducer Makes Imaging More Accessible
- Artificial Intelligence Detects Undiagnosed Liver Disease from Echocardiograms
- AI Model Significantly Enhances Low-Dose CT Capabilities
- Ultra-Low Dose CT Aids Pneumonia Diagnosis in Immunocompromised Patients
- AI Reduces CT Lung Cancer Screening Workload by Almost 80%
- Cutting-Edge Technology Combines Light and Sound for Real-Time Stroke Monitoring
- AI System Detects Subtle Changes in Series of Medical Images Over Time
- Global AI in Medical Diagnostics Market to Be Driven by Demand for Image Recognition in Radiology
- AI-Based Mammography Triage Software Helps Dramatically Improve Interpretation Process
- Artificial Intelligence (AI) Program Accurately Predicts Lung Cancer Risk from CT Images
- Image Management Platform Streamlines Treatment Plans
- AI Technology for Detecting Breast Cancer Receives CE Mark Approval
- Patient-Specific 3D-Printed Phantoms Transform CT Imaging
- Siemens and Sectra Collaborate on Enhancing Radiology Workflows
- Bracco Diagnostics and ColoWatch Partner to Expand Availability CRC Screening Tests Using Virtual Colonoscopy
- Mindray Partners with TeleRay to Streamline Ultrasound Delivery
- Philips and Medtronic Partner on Stroke Care
Expo
view channel
view channel
view channel
view channel
view channel
view channel
view channel
RadiographyMRIUltrasoundNuclear Medicine
Imaging ITIndustry News
Events
Advertise with Us
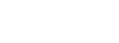
- Higher Chest X-Ray Usage Catches Lung Cancer Earlier and Improves Survival
- AI-Powered Mammograms Predict Cardiovascular Risk
- Generative AI Model Significantly Reduces Chest X-Ray Reading Time
- AI-Powered Mammography Screening Boosts Cancer Detection in Single-Reader Settings
- Photon Counting Detectors Promise Fast Color X-Ray Images
- AI-Powered MRI Technology Improves Parkinson’s Diagnoses
- Biparametric MRI Combined with AI Enhances Detection of Clinically Significant Prostate Cancer
- First-Of-Its-Kind AI-Driven Brain Imaging Platform to Better Guide Stroke Treatment Options
- New Model Improves Comparison of MRIs Taken at Different Institutions
- Groundbreaking New Scanner Sees 'Previously Undetectable' Cancer Spread
- Novel Radiotracer Identifies Biomarker for Triple-Negative Breast Cancer
- Innovative PET Imaging Technique to Help Diagnose Neurodegeneration
- New Molecular Imaging Test to Improve Lung Cancer Diagnosis
- Novel PET Technique Visualizes Spinal Cord Injuries to Predict Recovery
- Next-Gen Tau Radiotracers Outperform FDA-Approved Imaging Agents in Detecting Alzheimer’s
- Smart Ultrasound-Activated Immune Cells Destroy Cancer Cells for Extended Periods
- Tiny Magnetic Robot Takes 3D Scans from Deep Within Body
- High Resolution Ultrasound Speeds Up Prostate Cancer Diagnosis
- World's First Wireless, Handheld, Whole-Body Ultrasound with Single PZT Transducer Makes Imaging More Accessible
- Artificial Intelligence Detects Undiagnosed Liver Disease from Echocardiograms
- AI Model Significantly Enhances Low-Dose CT Capabilities
- Ultra-Low Dose CT Aids Pneumonia Diagnosis in Immunocompromised Patients
- AI Reduces CT Lung Cancer Screening Workload by Almost 80%
- Cutting-Edge Technology Combines Light and Sound for Real-Time Stroke Monitoring
- AI System Detects Subtle Changes in Series of Medical Images Over Time
- Global AI in Medical Diagnostics Market to Be Driven by Demand for Image Recognition in Radiology
- AI-Based Mammography Triage Software Helps Dramatically Improve Interpretation Process
- Artificial Intelligence (AI) Program Accurately Predicts Lung Cancer Risk from CT Images
- Image Management Platform Streamlines Treatment Plans
- AI Technology for Detecting Breast Cancer Receives CE Mark Approval
- Patient-Specific 3D-Printed Phantoms Transform CT Imaging
- Siemens and Sectra Collaborate on Enhancing Radiology Workflows
- Bracco Diagnostics and ColoWatch Partner to Expand Availability CRC Screening Tests Using Virtual Colonoscopy
- Mindray Partners with TeleRay to Streamline Ultrasound Delivery
- Philips and Medtronic Partner on Stroke Care