Expo
view channel
view channel
view channel
view channel
view channel
view channel
view channel
RadiographyMRIUltrasoundNuclear Medicine
Imaging ITIndustry News
Events
- Novel Breast Cancer Screening Technology Could Offer Superior Alternative to Mammogram
- Artificial Intelligence Accurately Predicts Breast Cancer Years Before Diagnosis
- AI-Powered Chest X-Ray Detects Pulmonary Nodules Three Years Before Lung Cancer Symptoms
- AI Model Identifies Vertebral Compression Fractures in Chest Radiographs
- Advanced 3D Mammography Detects More Breast Cancers
- Combining Imaging Techniques Could Enable Surgical Removal of Prostate Cancer Without Biopsy
- AI Supported Detection of Cerebral Multiple Sclerosis Lesions Cuts Radiologic Reporting Times
- AI Algorithm Detects 30% Of Breast Cancers Missed on MRI Scans
- Groundbreaking AI-Powered Software Significantly Enhances Brain MRI
- MRI Predicts Patient Outcomes and Tumor Recurrence in Rectal Cancer Patients
- New Imaging Agent to Drive Step-Change for Brain Cancer Imaging
- Portable PET Scanner to Detect Earliest Stages of Alzheimer’s Disease
- New Immuno-PET Imaging Technique Identifies Glioblastoma Patients Who Would Benefit from Immunotherapy
- PET Software Enhances Diagnosis and Monitoring of Alzheimer's Disease
- New Photon-Counting CT Technique Diagnoses Osteoarthritis Before Symptoms Develop
- Ultrasound Device Non-Invasively Improves Blood Circulation in Lower Limbs
- Wearable Ultrasound Device Provides Long-Term, Wireless Muscle Monitoring
- Ultrasound Can Identify Sources of Brain-Related Issues and Disorders Before Treatment
- New Guideline on Handling Endobronchial Ultrasound Transbronchial Needle Samples
- Groundbreaking Ultrasound-Guided Needle Insertion System Improves Medical Procedures
- Automated Multi-Patient CT Injection System Reduces Patient Set-Up Time and Streamlines Workflows
- Low-Dose CT Screening for Lung Cancer Can Benefit Heavy Smokers
- Non-Invasive Imaging Technique Accurately Detects Aggressive Kidney Cancer
- AI Algorithm Reduces Unnecessary Radiation Exposure in Traumatic Neuroradiological CT Scans
- New Solution Enhances AI-Based Quality Control and Diagnosis in Medical Imaging
- Global AI in Medical Diagnostics Market to Be Driven by Demand for Image Recognition in Radiology
- AI-Based Mammography Triage Software Helps Dramatically Improve Interpretation Process
- Artificial Intelligence (AI) Program Accurately Predicts Lung Cancer Risk from CT Images
- Image Management Platform Streamlines Treatment Plans
- AI Technology for Detecting Breast Cancer Receives CE Mark Approval
- Philips and Medtronic Partner on Stroke Care
- Siemens and Medtronic Enter into Global Partnership for Advancing Spine Care Imaging Technologies
- RSNA 2024 Technical Exhibits to Showcase Latest Advances in Radiology
- Bracco Collaborates with Arrayus on Microbubble-Assisted Focused Ultrasound Therapy for Pancreatic Cancer
- Innovative Collaboration to Enhance Ischemic Stroke Detection and Elevate Standards in Diagnostic Imaging
Expo
view channel
view channel
view channel
view channel
view channel
view channel
view channel
RadiographyMRIUltrasoundNuclear Medicine
Imaging ITIndustry News
Events
Advertise with Us
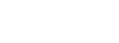
- Novel Breast Cancer Screening Technology Could Offer Superior Alternative to Mammogram
- Artificial Intelligence Accurately Predicts Breast Cancer Years Before Diagnosis
- AI-Powered Chest X-Ray Detects Pulmonary Nodules Three Years Before Lung Cancer Symptoms
- AI Model Identifies Vertebral Compression Fractures in Chest Radiographs
- Advanced 3D Mammography Detects More Breast Cancers
- Combining Imaging Techniques Could Enable Surgical Removal of Prostate Cancer Without Biopsy
- AI Supported Detection of Cerebral Multiple Sclerosis Lesions Cuts Radiologic Reporting Times
- AI Algorithm Detects 30% Of Breast Cancers Missed on MRI Scans
- Groundbreaking AI-Powered Software Significantly Enhances Brain MRI
- MRI Predicts Patient Outcomes and Tumor Recurrence in Rectal Cancer Patients
- New Imaging Agent to Drive Step-Change for Brain Cancer Imaging
- Portable PET Scanner to Detect Earliest Stages of Alzheimer’s Disease
- New Immuno-PET Imaging Technique Identifies Glioblastoma Patients Who Would Benefit from Immunotherapy
- PET Software Enhances Diagnosis and Monitoring of Alzheimer's Disease
- New Photon-Counting CT Technique Diagnoses Osteoarthritis Before Symptoms Develop
- Ultrasound Device Non-Invasively Improves Blood Circulation in Lower Limbs
- Wearable Ultrasound Device Provides Long-Term, Wireless Muscle Monitoring
- Ultrasound Can Identify Sources of Brain-Related Issues and Disorders Before Treatment
- New Guideline on Handling Endobronchial Ultrasound Transbronchial Needle Samples
- Groundbreaking Ultrasound-Guided Needle Insertion System Improves Medical Procedures
- Automated Multi-Patient CT Injection System Reduces Patient Set-Up Time and Streamlines Workflows
- Low-Dose CT Screening for Lung Cancer Can Benefit Heavy Smokers
- Non-Invasive Imaging Technique Accurately Detects Aggressive Kidney Cancer
- AI Algorithm Reduces Unnecessary Radiation Exposure in Traumatic Neuroradiological CT Scans
- New Solution Enhances AI-Based Quality Control and Diagnosis in Medical Imaging
- Global AI in Medical Diagnostics Market to Be Driven by Demand for Image Recognition in Radiology
- AI-Based Mammography Triage Software Helps Dramatically Improve Interpretation Process
- Artificial Intelligence (AI) Program Accurately Predicts Lung Cancer Risk from CT Images
- Image Management Platform Streamlines Treatment Plans
- AI Technology for Detecting Breast Cancer Receives CE Mark Approval
- Philips and Medtronic Partner on Stroke Care
- Siemens and Medtronic Enter into Global Partnership for Advancing Spine Care Imaging Technologies
- RSNA 2024 Technical Exhibits to Showcase Latest Advances in Radiology
- Bracco Collaborates with Arrayus on Microbubble-Assisted Focused Ultrasound Therapy for Pancreatic Cancer
- Innovative Collaboration to Enhance Ischemic Stroke Detection and Elevate Standards in Diagnostic Imaging