Expo
view channel
view channel
view channel
view channel
view channel
view channel
view channel
MRIUltrasoundNuclear MedicineGeneral/Advanced ImagingImaging ITIndustry News
Events
- AI Assistance Improves Breast-Cancer Screening by Reducing False Positives
- AI Could Boost Clinical Adoption of Chest DDR
- 3D Mammography Almost Halves Breast Cancer Incidence between Two Screening Tests
- AI Model Predicts 5-Year Breast Cancer Risk from Mammograms
- Deep Learning Framework Detects Fractures in X-Ray Images With 99% Accuracy
- World's First Whole-Body Ultra-High Field MRI Officially Comes To Market
- World's First Sensor Detects Errors in MRI Scans Using Laser Light and Gas
- Diamond Dust Could Offer New Contrast Agent Option for Future MRI Scans
- Combining MRI with PSA Testing Improves Clinical Outcomes for Prostate Cancer Patients
- PET/MRI Improves Diagnostic Accuracy for Prostate Cancer Patients
- New PET Agent Rapidly and Accurately Visualizes Lesions in Clear Cell Renal Cell Carcinoma Patients
- New Imaging Technique Monitors Inflammation Disorders without Radiation Exposure
- New SPECT/CT Technique Could Change Imaging Practices and Increase Patient Access
- New Radiotheranostic System Detects and Treats Ovarian Cancer Noninvasively
- AI System Automatically and Reliably Detects Cardiac Amyloidosis Using Scintigraphy Imaging
- Super-Resolution Imaging Technique Could Improve Evaluation of Cardiac Conditions
- First AI-Powered POC Ultrasound Diagnostic Solution Helps Prioritize Cases Based On Severity
- Largest Model Trained On Echocardiography Images Assesses Heart Structure and Function
- Groundbreaking Technology Enables Precise, Automatic Measurement of Peripheral Blood Vessels
- Deep Learning Advances Super-Resolution Ultrasound Imaging
- AI Predicts Cardiac Risk and Mortality from Routine Chest CT Scans
- Radiation Therapy Computed Tomography Solution Boosts Imaging Accuracy
- PET Scans Reveal Hidden Inflammation in Multiple Sclerosis Patients
- Artificial Intelligence Evaluates Cardiovascular Risk from CT Scans
- New AI Method Captures Uncertainty in Medical Images
- Global AI in Medical Diagnostics Market to Be Driven by Demand for Image Recognition in Radiology
- AI-Based Mammography Triage Software Helps Dramatically Improve Interpretation Process
- Artificial Intelligence (AI) Program Accurately Predicts Lung Cancer Risk from CT Images
- Image Management Platform Streamlines Treatment Plans
- AI Technology for Detecting Breast Cancer Receives CE Mark Approval
- Bayer and Google Partner on New AI Product for Radiologists
- Samsung and Bracco Enter Into New Diagnostic Ultrasound Technology Agreement
- IBA Acquires Radcal to Expand Medical Imaging Quality Assurance Offering
- International Societies Suggest Key Considerations for AI Radiology Tools
- Samsung's X-Ray Devices to Be Powered by Lunit AI Solutions for Advanced Chest Screening
Expo
view channel
view channel
view channel
view channel
view channel
view channel
view channel
MRIUltrasoundNuclear MedicineGeneral/Advanced ImagingImaging ITIndustry News
Events
Advertise with Us
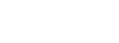
- AI Assistance Improves Breast-Cancer Screening by Reducing False Positives
- AI Could Boost Clinical Adoption of Chest DDR
- 3D Mammography Almost Halves Breast Cancer Incidence between Two Screening Tests
- AI Model Predicts 5-Year Breast Cancer Risk from Mammograms
- Deep Learning Framework Detects Fractures in X-Ray Images With 99% Accuracy
- World's First Whole-Body Ultra-High Field MRI Officially Comes To Market
- World's First Sensor Detects Errors in MRI Scans Using Laser Light and Gas
- Diamond Dust Could Offer New Contrast Agent Option for Future MRI Scans
- Combining MRI with PSA Testing Improves Clinical Outcomes for Prostate Cancer Patients
- PET/MRI Improves Diagnostic Accuracy for Prostate Cancer Patients
- New PET Agent Rapidly and Accurately Visualizes Lesions in Clear Cell Renal Cell Carcinoma Patients
- New Imaging Technique Monitors Inflammation Disorders without Radiation Exposure
- New SPECT/CT Technique Could Change Imaging Practices and Increase Patient Access
- New Radiotheranostic System Detects and Treats Ovarian Cancer Noninvasively
- AI System Automatically and Reliably Detects Cardiac Amyloidosis Using Scintigraphy Imaging
- Super-Resolution Imaging Technique Could Improve Evaluation of Cardiac Conditions
- First AI-Powered POC Ultrasound Diagnostic Solution Helps Prioritize Cases Based On Severity
- Largest Model Trained On Echocardiography Images Assesses Heart Structure and Function
- Groundbreaking Technology Enables Precise, Automatic Measurement of Peripheral Blood Vessels
- Deep Learning Advances Super-Resolution Ultrasound Imaging
- AI Predicts Cardiac Risk and Mortality from Routine Chest CT Scans
- Radiation Therapy Computed Tomography Solution Boosts Imaging Accuracy
- PET Scans Reveal Hidden Inflammation in Multiple Sclerosis Patients
- Artificial Intelligence Evaluates Cardiovascular Risk from CT Scans
- New AI Method Captures Uncertainty in Medical Images
- Global AI in Medical Diagnostics Market to Be Driven by Demand for Image Recognition in Radiology
- AI-Based Mammography Triage Software Helps Dramatically Improve Interpretation Process
- Artificial Intelligence (AI) Program Accurately Predicts Lung Cancer Risk from CT Images
- Image Management Platform Streamlines Treatment Plans
- AI Technology for Detecting Breast Cancer Receives CE Mark Approval
- Bayer and Google Partner on New AI Product for Radiologists
- Samsung and Bracco Enter Into New Diagnostic Ultrasound Technology Agreement
- IBA Acquires Radcal to Expand Medical Imaging Quality Assurance Offering
- International Societies Suggest Key Considerations for AI Radiology Tools
- Samsung's X-Ray Devices to Be Powered by Lunit AI Solutions for Advanced Chest Screening