Expo
view channel
view channel
view channel
view channel
view channel
view channel
view channel
Radiography
UltrasoundNuclear MedicineGeneral/Advanced ImagingImaging ITIndustry News
Events
- AI Method Accurately Predicts Breast Cancer Risk by Analyzing Multiple Mammograms
- Printable Organic X-Ray Sensors Could Transform Treatment for Cancer Patients
- Highly Sensitive, Foldable Detector to Make X-Rays Safer
- Novel Breast Cancer Screening Technology Could Offer Superior Alternative to Mammogram
- Artificial Intelligence Accurately Predicts Breast Cancer Years Before Diagnosis
- New Model Makes MRI More Accurate and Reliable
- New Scan Method Shows Effects of Treatment on Lung Function in Real Time
- Simple Scan Could Identify Patients at Risk for Serious Heart Problems
- Pioneering MRI Technique Detects Pre-Malignant Pancreatic Lesions for The First Time
- Portable MRI Shows Promise for Expanding Brain Imaging for Alzheimer’s Disease
- Combining Advanced Imaging Technologies Offers Breakthrough in Glioblastoma Treatment
- New Molecular Imaging Agent Accurately Identifies Crucial Cancer Biomarker
- New Scans Light Up Aggressive Tumors for Better Treatment
- AI Stroke Brain Scan Readings Twice as Accurate as Current Method
- AI Analysis of PET/CT Images Predicts Side Effects of Immunotherapy in Lung Cancer
- Automated Breast Ultrasound Provides Alternative to Mammography in Low-Resource Settings
- Transparent Ultrasound Transducer for Photoacoustic and Ultrasound Endoscopy to Improve Diagnostic Accuracy
- Wearable Ultrasound Patch Enables Continuous Blood Pressure Monitoring
- AI Image-Recognition Program Reads Echocardiograms Faster, Cuts Results Wait Time
- Ultrasound Device Non-Invasively Improves Blood Circulation in Lower Limbs
- New Technology Provides Coronary Artery Calcification Scoring on Ungated Chest CT Scans
- Deep Learning Model Accurately Diagnoses COPD Using Single Inhalation Lung CT Scan
- AI Model Reconstructs Sparse-View 3D CT Scan With Much Lower X-Ray Dose
- New Medical Scanner Identifies Brain Damage in Stroke Patients at Lower Magnetic Fields
- AI Tool Offers Opportunistic Screening for Heart Disease Using Repurposed CT Scans
- Global AI in Medical Diagnostics Market to Be Driven by Demand for Image Recognition in Radiology
- AI-Based Mammography Triage Software Helps Dramatically Improve Interpretation Process
- Artificial Intelligence (AI) Program Accurately Predicts Lung Cancer Risk from CT Images
- Image Management Platform Streamlines Treatment Plans
- AI Technology for Detecting Breast Cancer Receives CE Mark Approval
- Mindray Partners with TeleRay to Streamline Ultrasound Delivery
- Philips and Medtronic Partner on Stroke Care
- Siemens and Medtronic Enter into Global Partnership for Advancing Spine Care Imaging Technologies
- RSNA 2024 Technical Exhibits to Showcase Latest Advances in Radiology
- Bracco Collaborates with Arrayus on Microbubble-Assisted Focused Ultrasound Therapy for Pancreatic Cancer
Expo
view channel
view channel
view channel
view channel
view channel
view channel
view channel
Radiography
UltrasoundNuclear MedicineGeneral/Advanced ImagingImaging ITIndustry News
Events
Advertise with Us
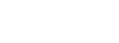
- AI Method Accurately Predicts Breast Cancer Risk by Analyzing Multiple Mammograms
- Printable Organic X-Ray Sensors Could Transform Treatment for Cancer Patients
- Highly Sensitive, Foldable Detector to Make X-Rays Safer
- Novel Breast Cancer Screening Technology Could Offer Superior Alternative to Mammogram
- Artificial Intelligence Accurately Predicts Breast Cancer Years Before Diagnosis
- New Model Makes MRI More Accurate and Reliable
- New Scan Method Shows Effects of Treatment on Lung Function in Real Time
- Simple Scan Could Identify Patients at Risk for Serious Heart Problems
- Pioneering MRI Technique Detects Pre-Malignant Pancreatic Lesions for The First Time
- Portable MRI Shows Promise for Expanding Brain Imaging for Alzheimer’s Disease
- Combining Advanced Imaging Technologies Offers Breakthrough in Glioblastoma Treatment
- New Molecular Imaging Agent Accurately Identifies Crucial Cancer Biomarker
- New Scans Light Up Aggressive Tumors for Better Treatment
- AI Stroke Brain Scan Readings Twice as Accurate as Current Method
- AI Analysis of PET/CT Images Predicts Side Effects of Immunotherapy in Lung Cancer
- Automated Breast Ultrasound Provides Alternative to Mammography in Low-Resource Settings
- Transparent Ultrasound Transducer for Photoacoustic and Ultrasound Endoscopy to Improve Diagnostic Accuracy
- Wearable Ultrasound Patch Enables Continuous Blood Pressure Monitoring
- AI Image-Recognition Program Reads Echocardiograms Faster, Cuts Results Wait Time
- Ultrasound Device Non-Invasively Improves Blood Circulation in Lower Limbs
- New Technology Provides Coronary Artery Calcification Scoring on Ungated Chest CT Scans
- Deep Learning Model Accurately Diagnoses COPD Using Single Inhalation Lung CT Scan
- AI Model Reconstructs Sparse-View 3D CT Scan With Much Lower X-Ray Dose
- New Medical Scanner Identifies Brain Damage in Stroke Patients at Lower Magnetic Fields
- AI Tool Offers Opportunistic Screening for Heart Disease Using Repurposed CT Scans
- Global AI in Medical Diagnostics Market to Be Driven by Demand for Image Recognition in Radiology
- AI-Based Mammography Triage Software Helps Dramatically Improve Interpretation Process
- Artificial Intelligence (AI) Program Accurately Predicts Lung Cancer Risk from CT Images
- Image Management Platform Streamlines Treatment Plans
- AI Technology for Detecting Breast Cancer Receives CE Mark Approval
- Mindray Partners with TeleRay to Streamline Ultrasound Delivery
- Philips and Medtronic Partner on Stroke Care
- Siemens and Medtronic Enter into Global Partnership for Advancing Spine Care Imaging Technologies
- RSNA 2024 Technical Exhibits to Showcase Latest Advances in Radiology
- Bracco Collaborates with Arrayus on Microbubble-Assisted Focused Ultrasound Therapy for Pancreatic Cancer