Expo
view channel
view channel
view channel
view channel
view channel
view channel
view channel
RadiographyMRIUltrasoundNuclear Medicine
Imaging ITIndustry News
Events
- AI Method Accurately Predicts Breast Cancer Risk by Analyzing Multiple Mammograms
- Printable Organic X-Ray Sensors Could Transform Treatment for Cancer Patients
- Highly Sensitive, Foldable Detector to Make X-Rays Safer
- Novel Breast Cancer Screening Technology Could Offer Superior Alternative to Mammogram
- Artificial Intelligence Accurately Predicts Breast Cancer Years Before Diagnosis
- Simple Scan Could Identify Patients at Risk for Serious Heart Problems
- Pioneering MRI Technique Detects Pre-Malignant Pancreatic Lesions for The First Time
- Portable MRI Shows Promise for Expanding Brain Imaging for Alzheimer’s Disease
- MRI-Based Imaging Technique Enables Rapid Assessment of Ovarian Cancer Subtypes and Treatment Response
- Enhanced Cardiovascular MRI Predicts Heart Risk in Children with Hypertrophic Cardiomyopathy
- AI Stroke Brain Scan Readings Twice as Accurate as Current Method
- AI Analysis of PET/CT Images Predicts Side Effects of Immunotherapy in Lung Cancer
- New Imaging Agent to Drive Step-Change for Brain Cancer Imaging
- Portable PET Scanner to Detect Earliest Stages of Alzheimer’s Disease
- New Immuno-PET Imaging Technique Identifies Glioblastoma Patients Who Would Benefit from Immunotherapy
- Wearable Ultrasound Patch Enables Continuous Blood Pressure Monitoring
- AI Image-Recognition Program Reads Echocardiograms Faster, Cuts Results Wait Time
- Ultrasound Device Non-Invasively Improves Blood Circulation in Lower Limbs
- Wearable Ultrasound Device Provides Long-Term, Wireless Muscle Monitoring
- Ultrasound Can Identify Sources of Brain-Related Issues and Disorders Before Treatment
- AI Model Reconstructs Sparse-View 3D CT Scan With Much Lower X-Ray Dose
- New Medical Scanner Identifies Brain Damage in Stroke Patients at Lower Magnetic Fields
- AI Tool Offers Opportunistic Screening for Heart Disease Using Repurposed CT Scans
- Lung Scans Detect Heart Disease in Patients Without Cardiac Symptoms
- AI Algorithms Accurately Predict Tumor Location and Size from Medical Images
- Global AI in Medical Diagnostics Market to Be Driven by Demand for Image Recognition in Radiology
- AI-Based Mammography Triage Software Helps Dramatically Improve Interpretation Process
- Artificial Intelligence (AI) Program Accurately Predicts Lung Cancer Risk from CT Images
- Image Management Platform Streamlines Treatment Plans
- AI Technology for Detecting Breast Cancer Receives CE Mark Approval
- Mindray Partners with TeleRay to Streamline Ultrasound Delivery
- Philips and Medtronic Partner on Stroke Care
- Siemens and Medtronic Enter into Global Partnership for Advancing Spine Care Imaging Technologies
- RSNA 2024 Technical Exhibits to Showcase Latest Advances in Radiology
- Bracco Collaborates with Arrayus on Microbubble-Assisted Focused Ultrasound Therapy for Pancreatic Cancer
Expo
view channel
view channel
view channel
view channel
view channel
view channel
view channel
RadiographyMRIUltrasoundNuclear Medicine
Imaging ITIndustry News
Events
Advertise with Us
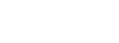
- AI Method Accurately Predicts Breast Cancer Risk by Analyzing Multiple Mammograms
- Printable Organic X-Ray Sensors Could Transform Treatment for Cancer Patients
- Highly Sensitive, Foldable Detector to Make X-Rays Safer
- Novel Breast Cancer Screening Technology Could Offer Superior Alternative to Mammogram
- Artificial Intelligence Accurately Predicts Breast Cancer Years Before Diagnosis
- Simple Scan Could Identify Patients at Risk for Serious Heart Problems
- Pioneering MRI Technique Detects Pre-Malignant Pancreatic Lesions for The First Time
- Portable MRI Shows Promise for Expanding Brain Imaging for Alzheimer’s Disease
- MRI-Based Imaging Technique Enables Rapid Assessment of Ovarian Cancer Subtypes and Treatment Response
- Enhanced Cardiovascular MRI Predicts Heart Risk in Children with Hypertrophic Cardiomyopathy
- AI Stroke Brain Scan Readings Twice as Accurate as Current Method
- AI Analysis of PET/CT Images Predicts Side Effects of Immunotherapy in Lung Cancer
- New Imaging Agent to Drive Step-Change for Brain Cancer Imaging
- Portable PET Scanner to Detect Earliest Stages of Alzheimer’s Disease
- New Immuno-PET Imaging Technique Identifies Glioblastoma Patients Who Would Benefit from Immunotherapy
- Wearable Ultrasound Patch Enables Continuous Blood Pressure Monitoring
- AI Image-Recognition Program Reads Echocardiograms Faster, Cuts Results Wait Time
- Ultrasound Device Non-Invasively Improves Blood Circulation in Lower Limbs
- Wearable Ultrasound Device Provides Long-Term, Wireless Muscle Monitoring
- Ultrasound Can Identify Sources of Brain-Related Issues and Disorders Before Treatment
- AI Model Reconstructs Sparse-View 3D CT Scan With Much Lower X-Ray Dose
- New Medical Scanner Identifies Brain Damage in Stroke Patients at Lower Magnetic Fields
- AI Tool Offers Opportunistic Screening for Heart Disease Using Repurposed CT Scans
- Lung Scans Detect Heart Disease in Patients Without Cardiac Symptoms
- AI Algorithms Accurately Predict Tumor Location and Size from Medical Images
- Global AI in Medical Diagnostics Market to Be Driven by Demand for Image Recognition in Radiology
- AI-Based Mammography Triage Software Helps Dramatically Improve Interpretation Process
- Artificial Intelligence (AI) Program Accurately Predicts Lung Cancer Risk from CT Images
- Image Management Platform Streamlines Treatment Plans
- AI Technology for Detecting Breast Cancer Receives CE Mark Approval
- Mindray Partners with TeleRay to Streamline Ultrasound Delivery
- Philips and Medtronic Partner on Stroke Care
- Siemens and Medtronic Enter into Global Partnership for Advancing Spine Care Imaging Technologies
- RSNA 2024 Technical Exhibits to Showcase Latest Advances in Radiology
- Bracco Collaborates with Arrayus on Microbubble-Assisted Focused Ultrasound Therapy for Pancreatic Cancer